Unlock Enterprise-Ready RAG with Cohere's Command-R+ Specialized Model
Unlock Enterprise-Ready RAG with Cohere's Command-R+ Specialized Model: Discover a powerful retrieval-augmented generation (RAG) model for enterprises, boasting high accuracy, low latency, and multilingual capabilities. Explore its impressive performance on key benchmarks.
January 24, 2025
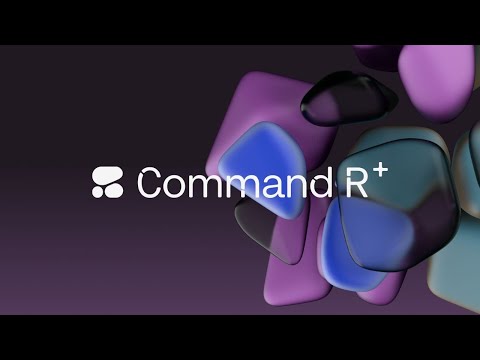
Unlock the power of Cohere's Command-R+ model, a specialized tool for Retrieval Augmented Generation (RAG) and beyond. Discover how this cutting-edge language model can enhance your enterprise-ready solutions, delivering accurate, verifiable information and mitigating hallucination. Explore its multilingual capabilities, impressive performance, and cost-effective pricing, making it a game-changer for industries like finance, HR, sales, marketing, and customer support.
Key Capabilities of Cohere's Command-R+ Model
Comparison to Other Large Language Models
Pricing and Accessibility
Hands-On Demonstration: Coral with Web Search and Coral with Documents
Conclusion
Key Capabilities of Cohere's Command-R+ Model
Key Capabilities of Cohere's Command-R+ Model
Cohere's Command-R+ model is a powerful retrieval-augmented generation (RAG) model designed for enterprise-ready applications. Here are the key capabilities of this model:
-
Accuracy on RAG Tasks: The Command-R+ model demonstrates strong accuracy on RAG tasks, outperforming other large language models like Mistral Large and GPT-4 Turbo.
-
Tool Usage: The model claims to outperform GPT-4 Turbo in tool usage, allowing it to effectively leverage external information sources to generate accurate and reliable responses.
-
Large Context Window: The model has a much larger context window of 128,000 tokens, enabling it to handle complex, multi-step queries and tasks.
-
Multilingual Support: The Command-R+ model supports 10 different languages, including English, French, Spanish, Italian, German, Portuguese, Japanese, Korean, Arabic, and Chinese, making it a versatile solution for enterprises serving customers in diverse regions.
-
Competitive Pricing: Compared to other large language models like Mistral Large and GPT-4 Turbo, the Command-R+ model is priced lower, offering a more cost-effective solution for enterprises.
-
Inline Citation: The model provides inline citations for its responses, helping to mitigate hallucination and improve the reliability of the information provided.
-
Optimized for Enterprise-Ready RAG: The Command-R+ model is specifically optimized for advanced RAG tasks, making it a suitable solution for finance, HR, sales, marketing, and customer support use cases.
Overall, the Command-R+ model from Cohere appears to be a compelling option for enterprises seeking a powerful, reliable, and cost-effective RAG solution that can handle a wide range of languages and use cases.
Comparison to Other Large Language Models
Comparison to Other Large Language Models
The Command R+ model from Cohere demonstrates impressive performance compared to other prominent large language models (LLMs) like Anthropic's GPT-4 Turbo and Anthropic's Mixlarge.
In terms of multilingual capabilities, the Command R+ model outperforms Anthropic's Mixlarge model by a significant margin and is very close to the performance of GPT-4 Turbo.
For retrieval-augmented generation (RAG) tasks, the Command R+ model shows a similar pattern, performing strongly against the competition.
Notably, Cohere claims the Command R+ model outperforms GPT-4 Turbo in tool usage, which could be a key differentiator for enterprise use cases.
Regarding pricing, the Command R+ model is priced lower than GPT-4 Turbo and Anthropic's Mixlarge, but higher than some other LLM providers. However, Cohere's focus on RAG and enterprise-ready reliability may justify the pricing for relevant use cases.
The model's multilingual support, spanning 10 languages including Arabic and Portuguese, sets it apart from many other LLMs that have more limited language coverage. This makes the Command R+ a compelling option for enterprises serving customers across diverse regions and languages.
Overall, the Command R+ model appears to be a strong performer, particularly for RAG tasks and enterprise applications, with competitive pricing and broad multilingual capabilities.
Pricing and Accessibility
Pricing and Accessibility
Coare's Command R+ model is priced lower than the Mistral Large and GPT-4 Turbo models, but higher than some other LLM providers. The company has focused on making the model accessible for enterprise use cases.
The pricing options for experimenting with the model include:
- Coral Chat: Directly chat with the model to generate responses.
- Coral with Web Search: Augment responses with web search capabilities.
- Coral with Documents: Perform retrieval-augmented generation on your own documents by uploading them in PDF or text format.
While the model weights are publicly available, Coare does not allow commercial use of the model outside of their API. This appears to be a strategic decision to maintain control and ensure the model is used for its intended enterprise-focused applications.
Overall, the pricing and accessibility of the Command R+ model seem tailored towards enterprises that require reliable, verifiable, and multilingual retrieval-augmented generation capabilities at scale.
Hands-On Demonstration: Coral with Web Search and Coral with Documents
Hands-On Demonstration: Coral with Web Search and Coral with Documents
In this section, we will explore the capabilities of the Cohere Command R+ model through two hands-on demonstrations: Coral with Web Search and Coral with Documents.
Coral with Web Search
We first tested the Coral with Web Search functionality. When asked "What is Jamba LLM?", the model provided a concise and informative response, citing relevant web sources to support the information. The inline citation feature allows users to easily verify the sources used to generate the response.
Additionally, the model suggested follow-up questions, demonstrating its ability to engage in a conversational flow and provide further insights on the topic.
Coral with Documents
Next, we tested the Coral with Documents functionality. We provided the model with a document and asked "What is instruction tuning?". The model generated a response that directly referenced the information in the document, highlighting the relevant section. This showcases the model's capability to perform retrieval-augmented generation, leveraging the provided documents to deliver a well-informed and accurate answer.
Similar to the Coral with Web Search, the model suggested follow-up questions, indicating its potential to engage in a deeper, more comprehensive discussion.
Overall, these hands-on demonstrations highlight the strong performance of the Cohere Command R+ model in retrieval-augmented generation tasks, its ability to provide inline citations, and its potential to enhance enterprise-level applications that require reliable and verifiable information.
Conclusion
Conclusion
The Cohere Command R+ model is a powerful and impressive language model that is specifically designed for retrieval-augmented generation (RAG) tasks. With its large 104 billion parameter size, multilingual support, and strong performance on benchmarks, it appears to be a compelling option for enterprises that require reliable and verifiable information across a variety of use cases.
The model's ability to provide inline citations and mitigate hallucination is particularly noteworthy, making it a suitable choice for applications in finance, HR, sales, marketing, and customer support. The pricing, while higher than some other providers, seems reasonable given the model's capabilities.
While the model is not open-source, Cohere's strategy of making the model weights publicly available for experimentation, while requiring the use of their API for commercial use, is an interesting approach. This allows users to explore the model's capabilities without the burden of hosting and maintaining the infrastructure.
Overall, the Cohere Command R+ model appears to be a strong contender in the enterprise-focused RAG space, and is worth considering for organizations with relevant use cases.
FAQ
FAQ