Kyutai's Groundbreaking VOICE AI Model Redefines Conversational AI
Kyutai's groundbreaking VOICE AI model, Moshi, redefines conversational AI with its ability to express over 70 emotions, speaking styles, and multilingual capabilities. This multimodal and multistream AI framework pushes the boundaries of real-time interactions, offering a seamless and human-like conversational experience.
July 20, 2024
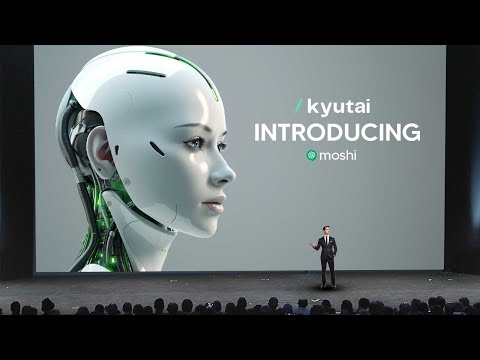
Discover the future of AI-powered conversations with Kyutai's groundbreaking "VOICE AI" technology. This cutting-edge model delivers lifelike interactions, seamless multimodal capabilities, and unparalleled emotional expression, redefining the way we engage with AI assistants.
Moshi's Impressive Capabilities: From Emotions to Accents
Overcoming Limitations of Current Voice AI Approaches
Breakthroughs in Moshi's Development: Multimodality, Multistream, and Adaptability
Moshi's TTS Engine and Voice Synthesis
Training Moshi: From Text-Only to Conversational AI
Running Moshi Locally on Device
Ensuring AI Safety with Moshi
Conclusion
Moshi's Impressive Capabilities: From Emotions to Accents
Moshi's Impressive Capabilities: From Emotions to Accents
Moshi is a remarkable AI model that can express a wide range of emotions and speaking styles. Its capabilities are truly impressive:
- Moshi can speak with over 70 different emotions, from whispering to sounding terrified, and can even impersonate a pirate or speak with a French accent.
- The model is able to respond in real-time, engaging in natural conversations and adapting its tone and language to the situation.
- Moshi's text-to-speech engine is highly advanced, generating lifelike audio that seamlessly blends emotion and personality.
- The model was trained on a diverse dataset, allowing it to handle a variety of topics and tasks, from reciting poetry to discussing current events.
- Moshi's multimodal nature, combining audio and textual outputs, enhances its ability to communicate effectively and provide comprehensive responses.
- The model's impressive performance was achieved through innovative training techniques, including the use of synthetic dialogues to fine-tune Moshi's conversational abilities.
Overall, Moshi represents a significant breakthrough in conversational AI, setting a new standard for natural, engaging, and emotionally expressive interactions.
Overcoming Limitations of Current Voice AI Approaches
Overcoming Limitations of Current Voice AI Approaches
The current approaches to voice AI have two main limitations that CAAI had to address in developing Moshi:
-
Latency: The complex pipeline of separate models induces a latency of 3-5 seconds between the user's input and the system's response. This can be extremely annoying for a live conversational experience.
-
Loss of Non-Textual Information: By going through a text-based bottleneck, the system loses all the non-textual information like emotion, tone, and communication cues present in the original speech.
To tackle these limitations, CAAI took a different approach. Instead of using a complex pipeline of separate models, they designed a single deep neural network-based "audio language model". This model is trained directly on annotated speech data, without the intermediate text representation.
By compressing the speech input into a compact "pseudo-word" representation, the audio language model can learn the patterns and structure of speech, similar to how text language models learn from text. This allows the model to generate responses that preserve the richness of the original speech, without the latency introduced by the text-based approach.
The result is a more natural, low-latency conversational experience that captures the full expressiveness of human speech.
Breakthroughs in Moshi's Development: Multimodality, Multistream, and Adaptability
Breakthroughs in Moshi's Development: Multimodality, Multistream, and Adaptability
The key breakthroughs in the development of Moshi, the advanced conversational AI model, are:
-
Multimodality: Moshi can not only listen and generate audio, but it also has textual thoughts that are displayed on the screen during the conversation. This allows it to leverage the efficiency and compactness of written text along with the richness of audio to provide better and faster responses.
-
Multistream: Moshi operates with two parallel audio streams, allowing it to speak and listen simultaneously. This enables more natural conversations with overlapping speech, interruptions, and seamless back-and-forth, making the interaction feel more human-like.
-
Adaptability: Moshi is not just a conversational speech AI model, but a flexible framework that can be adapted to various tasks and use cases. The team demonstrated Moshi's ability to engage in a discussion from the 1990s/2000s, showcasing its versatility and the potential to interact with data from different time periods.
These key breakthroughs, achieved by the Moshi team within just 6 months with a small team of 8 people, have enabled the creation of a highly realistic and responsive conversational AI system that can provide a truly immersive and natural interaction experience.
Moshi's TTS Engine and Voice Synthesis
Moshi's TTS Engine and Voice Synthesis
One of the most amazing things about Moshi is that it's not just some kind of AI model, but rather a text-to-speech engine that has over 70 different emotions that can be accessed. Using the recorded data, the team was able to train a text-to-speech engine that can support more than 70 different emotions or talking styles.
To showcase the capabilities of this TTS engine, the team played some generated audio samples. The samples demonstrated Moshi's ability to express a wide range of emotions, from whispering to singing, and even impersonating a pirate or speaking with a French accent. This showcases the impressive versatility and lifelike quality of Moshi's voice synthesis capabilities.
The team explained that this TTS engine was developed in-house, allowing them to fine-tune it specifically for Moshi's needs. By working with a voice artist named Alice, they were able to record various monologues and dialogues, which were then used to train the text-to-speech model. This approach ensures that Moshi has a consistent and natural-sounding voice across all interactions.
Overall, Moshi's TTS engine is a remarkable achievement, demonstrating the team's ability to push the boundaries of what's possible in voice synthesis and emotional expression. This capability, combined with Moshi's other breakthroughs, is what makes this model truly revolutionary and poised to change the landscape of conversational AI.
Training Moshi: From Text-Only to Conversational AI
Training Moshi: From Text-Only to Conversational AI
The key breakthroughs in training Moshi, the advanced conversational AI model, can be summarized as follows:
-
Multimodality: Moshi can not only generate audio, but also produce accompanying textual thoughts. This hybrid approach of combining audio and text allows for more efficient and effective training, leading to better responses.
-
Multistream Interaction: Moshi is capable of listening and speaking simultaneously, enabling natural conversational flow with overlapping speech, interruptions, and seamless back-and-forth exchanges, just like human conversations.
-
Synthetic Data Generation: To overcome the challenge of limited real-world conversational data, the team developed techniques to generate synthetic dialogues. This allowed them to fine-tune Moshi's conversational abilities beyond the initial text-only language model.
-
Voice Customization: By working with a professional voice artist, Alice, the team was able to imbue Moshi with a consistent and natural-sounding voice across interactions, further enhancing the user experience.
-
On-Device Deployment: The Moshi model is designed to be relatively small in size, enabling it to be deployed and run directly on devices, ensuring privacy and low-latency responses without the need for cloud connectivity.
-
Safety Considerations: Recognizing the potential for misuse, the team has implemented safeguards, such as audio watermarking and signature tracking, to detect and mitigate the generation of Moshi-like content for malicious purposes.
These advancements in training and deployment have allowed Moshi to achieve a remarkable level of conversational ability, seamlessly blending audio, text, and multimodal interaction to provide a truly immersive and natural user experience.
Running Moshi Locally on Device
Running Moshi Locally on Device
One of the key breakthroughs with Moshi is its ability to run locally on a device, without requiring an internet connection. This is a significant advancement, as it addresses concerns around privacy and latency that have plagued previous voice AI systems.
The team at CAAI demonstrated this capability by running Moshi on a standard MacBook Pro, with the internet connection disabled. They launched the Moshi application, and were able to engage in a real-time conversation with the AI assistant, without any noticeable lag or delay.
This on-device execution is made possible by the relatively small size of the Moshi model, which the team emphasized could be further compressed for deployment on mobile devices. By running the model locally, Moshi can provide a more seamless and private conversational experience, without the need to send audio data to a remote server.
The team also discussed the importance of safety and responsible development of such advanced AI systems. They outlined two key strategies to ensure the integrity of Moshi-generated content: online signature tracking and watermarking. These techniques allow for the detection of AI-generated audio, helping to mitigate the potential misuse of the technology.
Overall, the ability to run Moshi locally on a device is a significant milestone, demonstrating the team's commitment to delivering a high-performance, privacy-preserving conversational AI assistant. This advancement paves the way for more widespread adoption and integration of Moshi into various applications and use cases.
Ensuring AI Safety with Moshi
Ensuring AI Safety with Moshi
One of the last things that most people won't think about is of course the AI safety aspect. If you do have a model that is this quick and can respond with a remarkable degree of accuracy, we know that people could potentially use this for phishing campaigns or for other malicious activities. This is where they describe how they're going to safely identify Moshi content and ensure that this isn't a widespread problem.
Hello, I am from qAI. We are very serious about safety. One question in particular that we want to address is how to determine if an audio has been generated by Moshi or not. For this, we have considered two strategies:
-
Online Approach: We keep track of the audio that Moshi generates by extracting some signatures and putting them into a database of generated content. When presented with a new audio, we can extract a signature and check if it matches the database. If so, we know the audio is generated by Moshi.
-
Watermarking: We add some inaudible marks to the audio we generate, such that we can detect them with a specific detector. This allows us to identify Moshi-generated content.
These are active areas of research that are important, challenging, and interesting. We are committed to ensuring the safe and responsible development of Moshi to prevent any misuse or malicious activities.
Conclusion
Conclusion
This model, known as Moshi, represents a significant breakthrough in conversational AI. A few key highlights:
-
Moshi can express over 70 different emotions and speaking styles, from whispering to singing, allowing for highly natural and expressive interactions.
-
The model is multimodal, generating both audio and text simultaneously, which enhances the richness and coherence of the responses.
-
Moshi uses a novel "multistream" approach, allowing it to listen and speak concurrently, enabling more natural back-and-forth conversations with overlapping speech.
-
The model was trained efficiently using synthetic dialogues, overcoming the challenge of obtaining large amounts of real conversational data.
-
Importantly, the team has also addressed safety and security concerns, developing techniques to detect whether audio was generated by Moshi.
Overall, Moshi demonstrates remarkable capabilities that blur the line between human and machine interaction. This technology has the potential to transform how people engage with AI assistants, ushering in a new era of more natural, intelligent, and personalized conversations.
FAQ
FAQ