Balancing Smarts and Understandability: OpenAI's Approach to Explainable AI
Balancing AI Smarts and Understandability: OpenAI's Approach to Explainable AI. Discover how OpenAI is training AI models to be both highly capable and easily understandable, using a novel prover-verifier game approach.
December 22, 2024
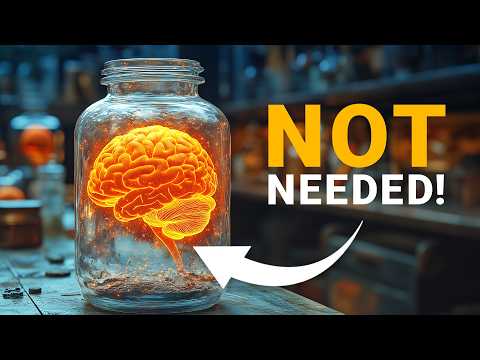
Discover how OpenAI's latest research challenges the notion that being smart is everything, and learn about their innovative approach to training AI models that are both highly capable and easily understandable. This blog post explores the fascinating insights from their groundbreaking paper, offering valuable lessons for the future of artificial intelligence.
The Challenge of Training Smarter AIs
The Game of Einstein and the Kid
The Power of Verifiability: Overcoming the Legibility Tax
Limitations and Future Potential
Conclusion
The Challenge of Training Smarter AIs
The Challenge of Training Smarter AIs
The paper highlights the challenge of training AI models that are both highly capable and easily understandable. While current AI systems can excel at solving complex problems, their solutions are often opaque and difficult for humans to comprehend.
The key insight is that as AI models become more powerful and accurate, they tend to become less interpretable. This "legibility tax" poses a significant challenge, as it limits the practical usefulness of these advanced AI systems.
To address this issue, the researchers propose a novel training approach inspired by the "prover-verifier" game. The idea is to train the AI model (the "prover") to not only solve difficult problems, but to also produce solutions that can be easily verified by a simpler "verifier" model, akin to a child.
This approach allows the AI to maintain its high performance while significantly improving the understandability of its solutions. Remarkably, the verifier model can be up to 1,000 times less capable than the prover, yet still be able to validate the solutions.
The paper demonstrates the effectiveness of this technique, showing how it can generate solutions that are both correct and easily interpretable, without sacrificing the model's overall capabilities. This represents an important step towards developing AI systems that are not only intelligent, but also transparent and accessible to human users.
The Game of Einstein and the Kid
The Game of Einstein and the Kid
The paper proposes a novel training approach to make AI models more understandable, even as they become more capable. The key idea is to play a "game" between two models: the "prover" model (akin to Einstein) that can solve difficult problems, and the "verifier" model (akin to a kid) that can easily verify the solutions.
The goal is to train the prover model to produce solutions that are easy for the verifier model to understand, even if the verifier is significantly less capable than the prover. This is achieved by having the prover model optimize not just for correctness, but also for the verifier's ability to follow the solution.
Remarkably, the paper shows that this approach can work even when the verifier model is up to 1000 times less capable than the prover. The verifier model acts as a "lie detector", ensuring that the prover model does not simply hand over an incorrect solution that the verifier cannot detect.
The main result is that this technique allows for models to become smarter without sacrificing understandability, a trade-off that is often observed in traditional AI training. This breakthrough has the potential to make advanced AI systems more accessible and transparent, with meaningful impacts across various domains.
The Power of Verifiability: Overcoming the Legibility Tax
The Power of Verifiability: Overcoming the Legibility Tax
The key insight of this new OpenAI paper is that while AI models can be trained to achieve high correctness, this often comes at the cost of poor understandability. However, the researchers propose a novel technique to overcome this "legibility tax" by leveraging the concept of verifiability.
The core idea is to train the AI model in a game-like setting, where a "prover" model (akin to Einstein) solves difficult problems, and a "verifier" model (akin to a child) is tasked with verifying the solutions. Remarkably, the verifier can be up to 1,000 times less capable than the prover, yet the game still works effectively.
This approach encourages the prover model to generate solutions that are not only correct but also easy for the verifier to understand and validate. By introducing the possibility of the verifier detecting lies or mistakes, the prover is incentivized to produce more legible and transparent solutions.
The results are quite impressive, as the researchers demonstrate that this technique can improve the understandability of the AI's solutions without sacrificing its overall performance. This represents a significant advancement, as it allows for the development of highly capable AI systems that are also more interpretable and accessible to human users.
While the technique currently works best in the domain of language, especially in the highly formalized context of mathematics, the researchers note that it remains unclear how it could be applied to other domains, such as image processing. Nonetheless, this work represents an important step towards creating AI systems that are not only intelligent but also more transparent and trustworthy.
Limitations and Future Potential
Limitations and Future Potential
While the proposed technique shows promising results in improving the understandability of AI models, particularly in the domain of language and mathematics, the authors acknowledge that it may have limitations in other domains, such as images. The highly formalized nature of mathematics makes it well-suited for this approach, but it is unclear how it could be applied to more complex and less structured domains.
The authors note that the technique works well within the language domain, but further research is needed to explore its potential in other areas. As the field of AI continues to advance, the ability to create models that are not only highly capable but also easily interpretable and understandable will become increasingly important. The work presented in this paper represents an important step in that direction, but there is still much to be explored and discovered.
Conclusion
Conclusion
The new OpenAI paper presents a remarkable approach to training AI models that are not only highly capable but also more understandable. By introducing a "prover-verifier" game, where a powerful "prover" model (akin to Einstein) solves complex problems and a less capable "verifier" model (akin to a child) can easily validate the solutions, the researchers have found a way to create AI systems that maintain their performance while becoming more legible and interpretable.
The key insight is that as AI models become more sophisticated, they often sacrifice understandability in pursuit of raw capability. This paper demonstrates that it is possible to overcome this trade-off, allowing for the development of highly capable AI systems that can also provide clear and accessible explanations of their solutions.
While the technique is currently most effective in the domain of language, especially mathematics, the potential implications of this work are far-reaching. By making AI systems more transparent and understandable, this approach could have a meaningful impact on a wide range of applications, from scientific research to decision-making processes, ultimately enhancing the integration of AI into our lives.
FAQ
FAQ