We have tested a variety of AI Knowledge Graph tools and services and selected the best ones for you.
Here we are listing the top 15 AI Knowledge Graph tools that we recommend.
DiveDeck.AI
StoicGPT
Famy - AI For Education (Gemini Pro)
frontiermodelforum.org
YouApp
gnshealthcare.com
promptengineering.org
wizenoze.com
charisma.ai
Neurture
Learnex AI
Future Proof
IYO AI - Talk to AI iOS App
AI Geometric
Zainii.AI
AI Knowledge Graph Use Cases
AI Knowledge Graph Use Cases
- #1
Creating a comprehensive knowledge graph for a specific industry or niche to help businesses better understand their market and competitors.
- #2
Utilizing AI to automatically update and expand knowledge graphs with the latest information and trends in a particular field.
- #3
Leveraging knowledge graphs to improve search engine optimization by providing search engines with structured data about entities and their relationships.
- #4
Using AI-powered knowledge graphs to enhance personalized recommendations for customers based on their preferences and behavior.
- #5
Implementing a knowledge graph to improve internal knowledge management within an organization by linking relevant information and resources.
What is a knowledge graph and how does it relate to artificial intelligence (AI)?
What is a knowledge graph and how does it relate to artificial intelligence (AI)?
A knowledge graph is a structured representation of knowledge that connects various entities, their properties, and the relationships between them. In the context of AI, knowledge graphs play a crucial role in enabling intelligent reasoning, natural language processing, and knowledge-powered decision making.
By modeling real-world concepts, entities, and their relationships, knowledge graphs provide a semantic foundation for AI systems to understand and reason about complex domains. They help AI models to better comprehend the context and meaning behind data, leading to more accurate and contextual outputs.
What are the key components and features of a state-of-the-art knowledge graph?
What are the key components and features of a state-of-the-art knowledge graph?
A state-of-the-art knowledge graph typically includes the following key components and features:
- Comprehensive Entity Representation: The knowledge graph should contain a diverse and extensive set of entities, including people, organizations, locations, events, concepts, and more, each with detailed attributes and properties.
- Robust Relationship Modeling: The knowledge graph should accurately capture the various types of relationships between entities, such as hierarchical, associative, and causal relationships, to enable complex reasoning and inference.
- Semantic Enrichment: The knowledge graph should incorporate semantic annotations and ontologies to provide deeper understanding of the meaning and context of the entities and their relationships.
- Continuous Learning and Evolution: The knowledge graph should have the ability to continuously expand and update its knowledge by ingesting new data sources and incorporating the latest information.
- Multimodal Integration: The knowledge graph should integrate and link various data modalities, such as text, images, and structured data, to provide a more comprehensive and cross-referential understanding of the entities and their connections.
- Explainability and Interpretability: The knowledge graph should support the explanation of its reasoning and decision-making processes, making the system's outputs more transparent and trustworthy.
How can a knowledge graph-powered AI system be used in various real-world applications?
How can a knowledge graph-powered AI system be used in various real-world applications?
Knowledge graph-powered AI systems can be leveraged in a wide range of real-world applications:
- Intelligent Search and Recommendations: By understanding the semantic relationships between entities, a knowledge graph-powered AI system can enhance search capabilities, provide more personalized and contextual recommendations, and enable exploratory discovery.
- Question Answering and Conversational AI: A knowledge graph can enable AI assistants to answer complex, context-aware questions by leveraging the structured knowledge and reasoning capabilities.
- Fraud Detection and Risk Management: Knowledge graphs can help identify patterns, relationships, and anomalies in data to detect fraudulent activities and assess risks more effectively.
- Biomedical Research and Drug Discovery: Knowledge graphs can integrate and connect diverse biomedical data, enabling AI systems to uncover new insights, identify potential drug targets, and accelerate the drug discovery process.
- Smart City and Infrastructure Planning: Knowledge graphs can model the complex interdependencies within a city's infrastructure, transportation systems, and urban planning to support more informed decision-making and optimization.
- Personalized Education and Training: By understanding the learner's knowledge, skills, and learning preferences, a knowledge graph-powered AI system can deliver personalized educational content and adaptive learning experiences.
What are the key technical challenges and considerations in building a state-of-the-art knowledge graph?
What are the key technical challenges and considerations in building a state-of-the-art knowledge graph?
Building a state-of-the-art knowledge graph presents several technical challenges and considerations:
- Data Integration and Curation: Integrating diverse data sources, resolving entity disambiguation, and ensuring data quality and consistency are critical for constructing a comprehensive and reliable knowledge graph.
- Scalable Knowledge Representation: Developing efficient and scalable knowledge representation models to handle the growing volume and complexity of entities, relationships, and attributes within the knowledge graph.
- Automated Knowledge Extraction: Designing robust techniques for automatically extracting and inferring knowledge from unstructured and semi-structured data sources, such as text, images, and web pages.
- Contextual Reasoning and Inference: Enabling the knowledge graph to perform contextual reasoning and inference by leveraging advanced language understanding, commonsense reasoning, and probabilistic models.
- Continuous Learning and Evolution: Implementing mechanisms for the knowledge graph to continuously expand, update, and refine its knowledge base as new information becomes available.
- Multimodal Integration: Integrating and aligning different data modalities, such as text, images, and structured data, within the knowledge graph to provide a more comprehensive understanding.
- Explainability and Trustworthiness: Developing approaches to make the knowledge graph-powered AI systems more transparent, interpretable, and trustworthy, allowing users to understand the reasoning behind the system's outputs.
How can knowledge graph-powered AI systems be evaluated and benchmarked?
How can knowledge graph-powered AI systems be evaluated and benchmarked?
Evaluating and benchmarking knowledge graph-powered AI systems involves several key considerations:
- Task-specific Evaluation: Defining and measuring the system's performance on specific application-oriented tasks, such as question answering, entity linking, relation extraction, and inference.
- Knowledge Graph Quality Metrics: Assessing the knowledge graph's coverage, accuracy, completeness, and consistency through metrics like entity and relation coverage, link prediction accuracy, and ontology alignment.
- Reasoning and Inference Capabilities: Evaluating the system's ability to perform complex reasoning, draw logical inferences, and generate contextually relevant outputs.
- Multimodal Integration and Alignment: Assessing the system's capacity to effectively integrate and align knowledge from different data modalities, such as text, images, and structured data.
- Explainability and Interpretability: Measuring the system's transparency and the ability to explain its reasoning and decision-making processes to users.
- User-centric Metrics: Incorporating end-user feedback, satisfaction, and trust as part of the evaluation process to ensure the system's practical usefulness and user-friendliness.
- Benchmarking Against Established Datasets: Comparing the system's performance against well-known benchmarks and datasets, such as those used in the annual Knowledge Graph Conference (KGC) and the Semantic Web Challenge.
- Continuous Evaluation and Monitoring: Establishing mechanisms for ongoing evaluation and monitoring of the knowledge graph-powered AI system's performance, robustness, and adaptability to evolving user needs and data landscapes.
- DiveDeck.AI
- DiveDeck.AI
- StoicGPT
- StoicGPT
- Famy - AI For Education (Gemini Pro)
- Famy - AI For Education (Gemini Pro)
- frontiermodelforum.org
- frontiermodelforum.org
- YouApp
- YouApp
- gnshealthcare.com
- gnshealthcare.com
- promptengineering.org
- promptengineering.org
- wizenoze.com
- wizenoze.com
- charisma.ai
- charisma.ai
- Neurture
- Neurture
- Learnex AI
- Learnex AI
- Future Proof
- Future Proof
- IYO AI - Talk to AI iOS App
- IYO AI - Talk to AI iOS App
- AI Geometric
- AI Geometric
- Zainii.AI
- Zainii.AI
Example of AI Knowledge Graph Tools
Example of AI Knowledge Graph Tools
Scite
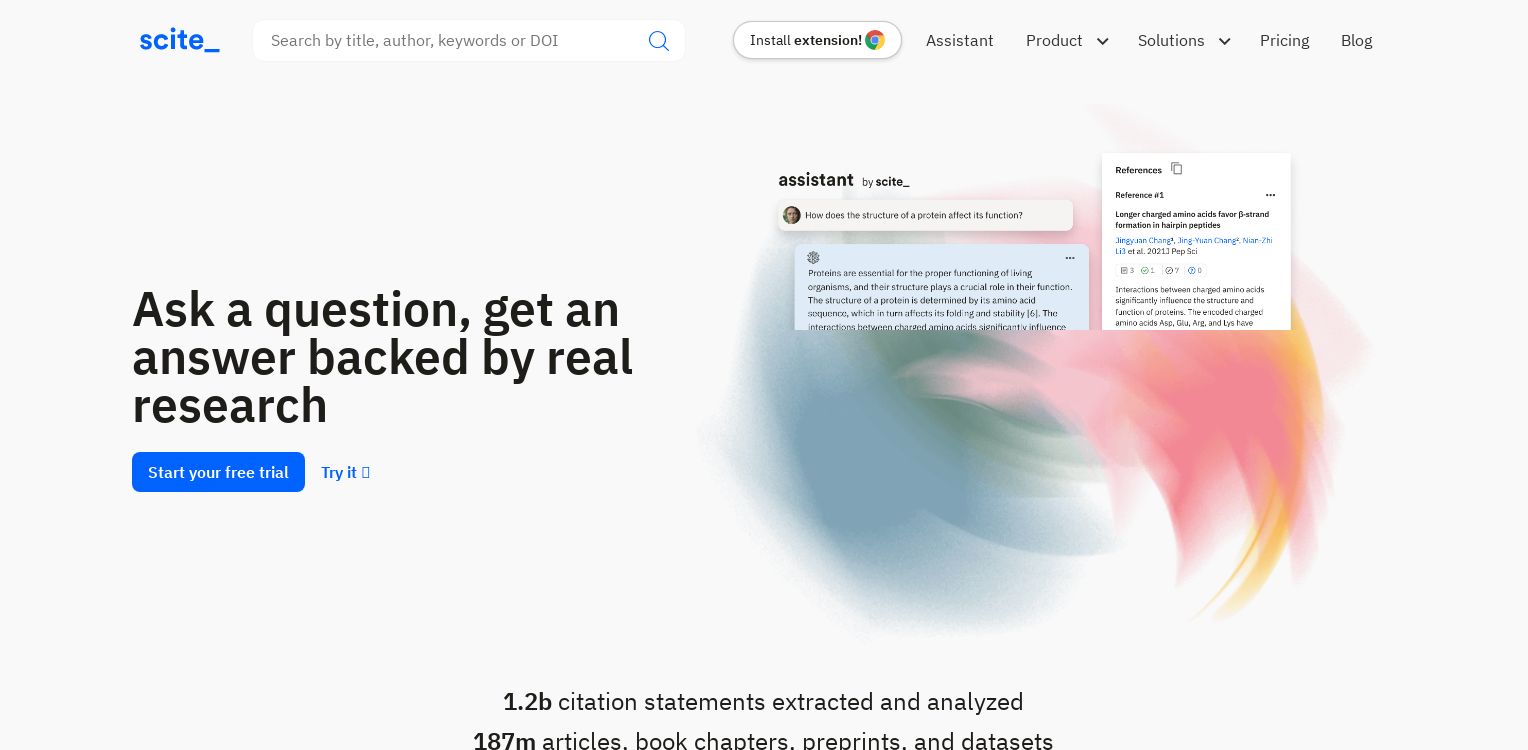
Scite is an AI-powered platform that helps researchers discover and evaluate scientific articles through the use of "Smart Citations" - citations that provide context and indicate whether the cited publication provides supporting or contrasting evidence for a particular claim.
Conclusion
Conclusion
In conclusion, the AI Knowledge Graph tools listed above are the best in their class. They offer a wide range of features and functionalities that cater to different needs and preferences. Whether you're looking for a tool to streamline your workflow, enhance your productivity, or drive innovation, these tools have got you covered. We recommend exploring each tool further, taking advantage of free trials or demos, and gathering feedback from your team to make an informed decision. By leveraging the capabilities of these cutting-edge tools, you can unlock new opportunities, drive growth, and stay ahead in today's competitive landscape.