מהפכת התמחות במודלים: כוחה של כוונון פרומפט
שנה את מודל ה-AI שלך באמצעות כוונון הפרומפט! גלה כיצד טכניקה יעילה זו יכולה להתמחות במודלי שפה גדולים ללא נתונים נרחבים או הדרכה מחדש. חקור את הכוח של פרומפטים רכים ואת היתרונות שלהם על פני כוונון מסורתי ותכנון פרומפט. שחרר אפשרויות חדשות בלמידה רב-משימתית והסתגלות מתמשכת.
25 בינואר 2025
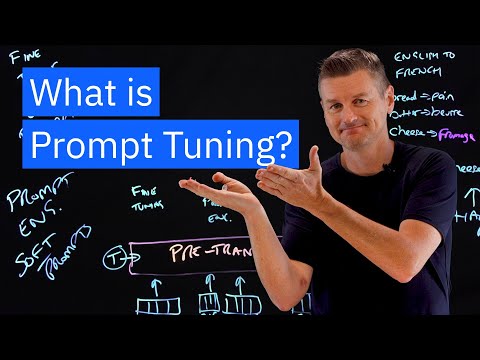
כוונון פרומפט הוא טכניקה עוצמתית המאפשרת לחברות עם נתונים מוגבלים להתאים מודלי שפה גדולים למשימות מתמחות ביעילות. פוסט הבלוג הזה חוקר כיצד פועל כוונון פרומפט, יתרונותיו על פני כוונון מסורתי ותכנון פרומפט, ויישומים מבטיחים בתחומים כמו למידה רב-משימתית ולמידה מתמשכת.
כוח הכוונון המהיר: התאמת דגמי שפה גדולים למשימות ייעודיות
כוונון הכוונון לעומת כוונון מדויק: התאמת יעילה של דגם
הנדסת הכוונון: אמנות יצירת כוונונים מותאמים אישית
כוונונים רכים: כוונונים מופקים על ידי AI לביצועים משופרים
כוונון הכוונון בפעולה: למידה רב-משימתית ולמידה מתמשכת
מסקנה
כוח הכוונון המהיר: התאמת דגמי שפה גדולים למשימות ייעודיות
כוח הכוונון המהיר: התאמת דגמי שפה גדולים למשימות ייעודיות
דוגמאות למודלי שפה גדולים כמו ChatGPT הם מודלי בסיס - מודלים גדולים ושימושיים רב-פעמיים שהוכשרו על כמויות עצומות של ידע מהאינטרנט. מודלים אלה גמישים מאוד, מסוגלים לנתח מסמכים משפטיים או לכתוב שיר על קבוצת כדורגל.
然而, 为了提高预训练的大型语言模型在专门任务上的性能, 一种更简单、更节能的技术已经出现: prompt tuning. Prompt tuning允许拥有有限数据的公司定制庞大的模型来执行非常狭窄的任务, 而无需收集数千个标记的示例来进行微调。
In prompt tuning, the best cues or front-end prompts are fed to the AI model to provide task-specific context. These prompts can be extra words introduced by humans or more commonly, AI-generated numerical embeddings introduced into the model's embedding layer to guide the model towards the desired decision or prediction.
Prompt engineering, the task of developing prompts that guide a large language model to perform specialized tasks, is an exciting field. However, AI-generated "soft" prompts have been shown to outperform human-engineered "hard" prompts, as they can distill knowledge from the larger model and act as a substitute for additional training data.
While prompt tuning offers advantages over fine-tuning and prompt engineering, it also has a drawback - its lack of interpretability. The AI-discovered prompts optimized for a given task are often opaque, similar to the opacity of deep learning models themselves.
In summary, prompt tuning is a game-changing technique that allows for faster and more cost-effective adaptation of large language models to specialized tasks, making it a valuable tool in areas like multitask learning and continual learning.
כוונון הכוונון לעומת כוונון מדויק: התאמת יעילה של דגם
כוונון הכוונון לעומת כוונון מדויק: התאמת יעילה של דגם
Fine-tuning is a method where a pre-trained model is supplemented with a large number of labeled examples specific to the target task. This allows the model to adapt and specialize for the task at hand. In contrast, prompt tuning is a simpler and more efficient technique that introduces task-specific context through prompts, without the need for extensive labeled data.
Prompt engineering involves manually crafting prompts that guide the pre-trained model to perform a specialized task. These "hard prompts" can be effective, but they require human effort and expertise. Soft prompts, on the other hand, are AI-generated embeddings that are optimized to steer the model towards the desired output. Soft prompts have been shown to outperform human-engineered prompts, making prompt tuning a more powerful and scalable approach.
The key advantage of prompt tuning is its efficiency. By leveraging the knowledge already captured in the pre-trained model and fine-tuning only the prompt, prompt tuning can adapt the model to specialized tasks much faster and with fewer resources than traditional fine-tuning. This makes it particularly useful for tasks that require quick adaptation, such as in multi-task learning or continual learning scenarios.
However, the downside of prompt tuning is its lack of interpretability. The AI-generated soft prompts are opaque, making it difficult to understand why the model chose a particular prompt. This contrasts with the transparency of human-engineered prompts in prompt engineering.
In summary, prompt tuning is a powerful and efficient technique for adapting pre-trained models to specialized tasks, outperforming traditional fine-tuning methods. While it may come at the cost of interpretability, the advantages of prompt tuning make it a valuable tool in the AI practitioner's arsenal.
הנדסת הכוונון: אמנות יצירת כוונונים מותאמים אישית
הנדסת הכוונון: אמנות יצירת כוונונים מותאמים אישית
Prompt engineering is the task of developing prompts that guide a large language model (LLM) to perform specialized tasks. Unlike fine-tuning, which requires gathering and labeling large datasets, prompt engineering allows companies with limited data to tailor a massive model to a narrow task.
The key to prompt engineering is crafting the right prompts. These prompts can be as simple as a few words or as complex as a multi-sentence instruction. The prompts provide task-specific context, guiding the LLM to retrieve the appropriate response from its vast memory.
For example, to train an LLM as an English-to-French translator, a prompt might start with "Translate the following English words to French:" followed by a few example translations. This primes the model to perform the desired task.
While human-engineered prompts, known as "hard prompts," can be effective, AI-generated "soft prompts" have been shown to outperform them. These soft prompts are unrecognizable to the human eye, consisting of embeddings or strings of numbers that distill knowledge from the larger model.
The advantage of soft prompts is their ability to adapt to complex tasks without the need for extensive human engineering. However, this comes at the cost of interpretability, as the AI-discovered prompts can be opaque, much like the inner workings of deep learning models themselves.
In summary, prompt engineering and prompt tuning are powerful techniques that allow for the efficient adaptation of large language models to specialized tasks, offering a more cost-effective alternative to traditional fine-tuning approaches.
כוונונים רכים: כוונונים מופקים על ידי AI לביצועים משופרים
כוונונים רכים: כוונונים מופקים על ידי AI לביצועים משופרים
Prompt tuning is a powerful technique that allows companies with limited data to tailor massive language models to specialized tasks. Unlike fine-tuning, which requires gathering and labeling thousands of examples, prompt tuning relies on AI-generated "soft prompts" to guide the model's output.
These soft prompts are unrecognizable to the human eye, consisting of numerical embeddings that distill knowledge from the larger model. They can be high-level or task-specific, acting as a substitute for additional training data and effectively guiding the model towards the desired output.
One key advantage of soft prompts is that they have been shown to outperform human-engineered "hard prompts" in many cases. While hard prompts require manual prompt engineering, soft prompts are automatically generated by the AI, optimizing for the given task.
However, the downside of soft prompts is their lack of interpretability. Unlike human-designed prompts, the AI-generated embeddings are opaque, making it difficult to understand why the model chose those specific prompts. This trade-off between performance and interpretability is a common challenge in deep learning models.
Despite this limitation, prompt tuning with soft prompts is proving to be a game-changer in various applications, such as multitask learning and continual learning. By allowing for faster and more cost-effective model adaptation, prompt tuning is making it easier to find and fix problems in specialized tasks.
כוונון הכוונון בפעולה: למידה רב-משימתית ולמידה מתמשכת
כוונון הכוונון בפעולה: למידה רב-משימתית ולמידה מתמשכת
Prompt tuning has emerged as a game-changing technique in various areas, particularly in multitask learning and continual learning.
In multitask learning, where models need to quickly switch between tasks, researchers are finding ways to create universal prompts that can be easily recycled. Techniques like multitask prompt tuning allow the model to be adapted swiftly and at a fraction of the cost of retraining.
Prompt tuning is also showing promise in the field of continual learning, where AI models need to learn new tasks and concepts without forgetting the old ones. Essentially, prompt tuning allows you to adapt your model to specialized tasks faster than fine-tuning and prompt engineering, making it easier to find and fix problems.
The key advantage of prompt tuning is its ability to leverage the power of pre-trained large language models while tailoring them to specific tasks. By introducing AI-generated soft prompts, the model can be guided towards the desired output without the need for extensive fine-tuning or manual prompt engineering.
While prompt tuning may lack the interpretability of human-engineered prompts, its efficiency and effectiveness in adapting models to specialized tasks make it a valuable tool in the AI researcher's arsenal.
מסקנה
מסקנה
The emergence of prompt tuning as an alternative to fine-tuning and prompt engineering has significant implications for the field of large language models. Prompt tuning allows companies with limited data to tailor massive models to specialized tasks, without the need for gathering thousands of labeled examples required for fine-tuning.
The key advantage of prompt tuning is the use of AI-generated "soft prompts" that are optimized for the desired task. These soft prompts, represented as embeddings or strings of numbers, outperform human-engineered "hard prompts" in guiding the model towards the desired output.
While prompt tuning lacks the interpretability of human-designed prompts, it offers several benefits. It enables faster adaptation of models to new tasks, making it particularly useful for multitask learning and continual learning scenarios. Additionally, the cost-effectiveness of prompt tuning compared to retraining models from scratch makes it an attractive option for organizations looking to specialize their large language models.
As the field of prompt-based learning continues to evolve, the role of prompt engineers may diminish, as AI-generated soft prompts become increasingly sophisticated and effective. However, the fundamental principles of prompt engineering, such as understanding how to craft prompts to guide model behavior, remain valuable and will likely continue to play a role in the development of advanced language models.
שאלות נפוצות
שאלות נפוצות